Drowsiness 2.0 - Alerting drivers before it is too late
Drowsy driving is a serious risk for commercial fleets. LightMetrics’ Drowsiness 2.0 uses AI to detect early signs of driver fatigue by analyzing eye openness, blink rate, and duration. This upgrade delivers real-time alerts to drivers, helping prevent accidents and reducing fleet-related costs. By addressing fatigue before it becomes critical, Drowsiness 2.0 enhances driver safety and protects fleet assets, ensuring drivers stay alert and safe on the road.
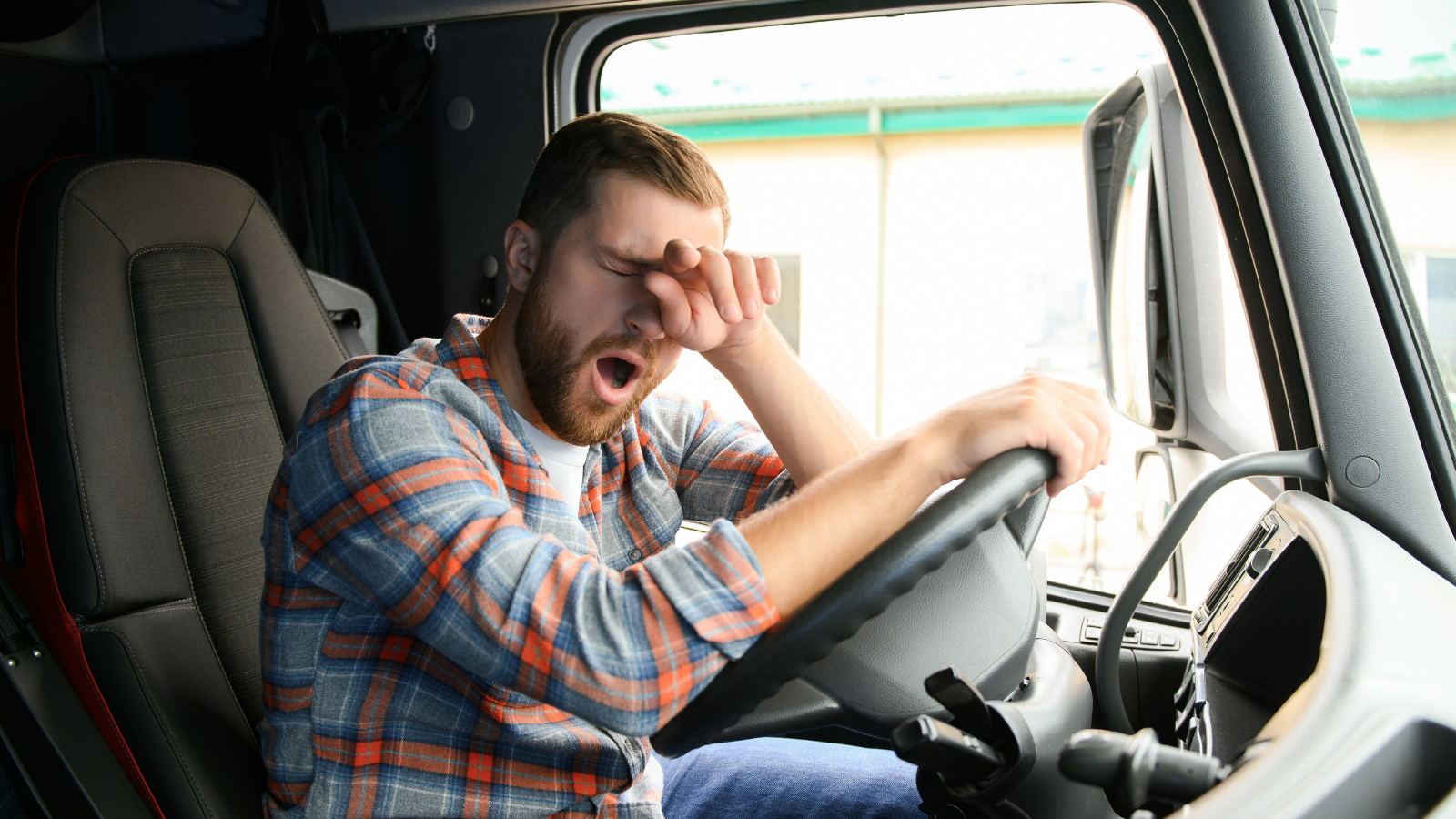
Drowsy driving is one of the most dangerous, yet often overlooked, risky driving behaviors, especially in commercial fleets. Fatigue impairs a driver's reaction time, attention, and decision-making, significantly increasing the likelihood of accidents. According to the National Safety Council, drowsy driving is responsible for approximately 100,000 crashes, 71,000 injuries, and 1,550 fatalities annually in the U.S. alone. The National Highway Traffic Safety Administration (NHTSA) estimates that fatigue-related crashes cost the U.S. economy over $109 billion each year, excluding property damage. For commercial fleets, these incidents lead to higher insurance premiums, lost productivity, and increased operational costs, making it crucial to address drowsiness with real-time detection and alert systems.
Combating drowsy driving and fatigue was a key reason behind the introduction of hours-of-service regulation in the US for truck drivers (and equivalent legislations in other countries).
LightMetrics introduced alerts for drowsiness and fatigue more than a year back - this was based on AI detecting eyes being closed or nearly closed.
We are excited to introduce Drowsiness 2.0, a significant update to the earlier algorithm - the improvement is thanks to all our partners globally, the data makes the system better.
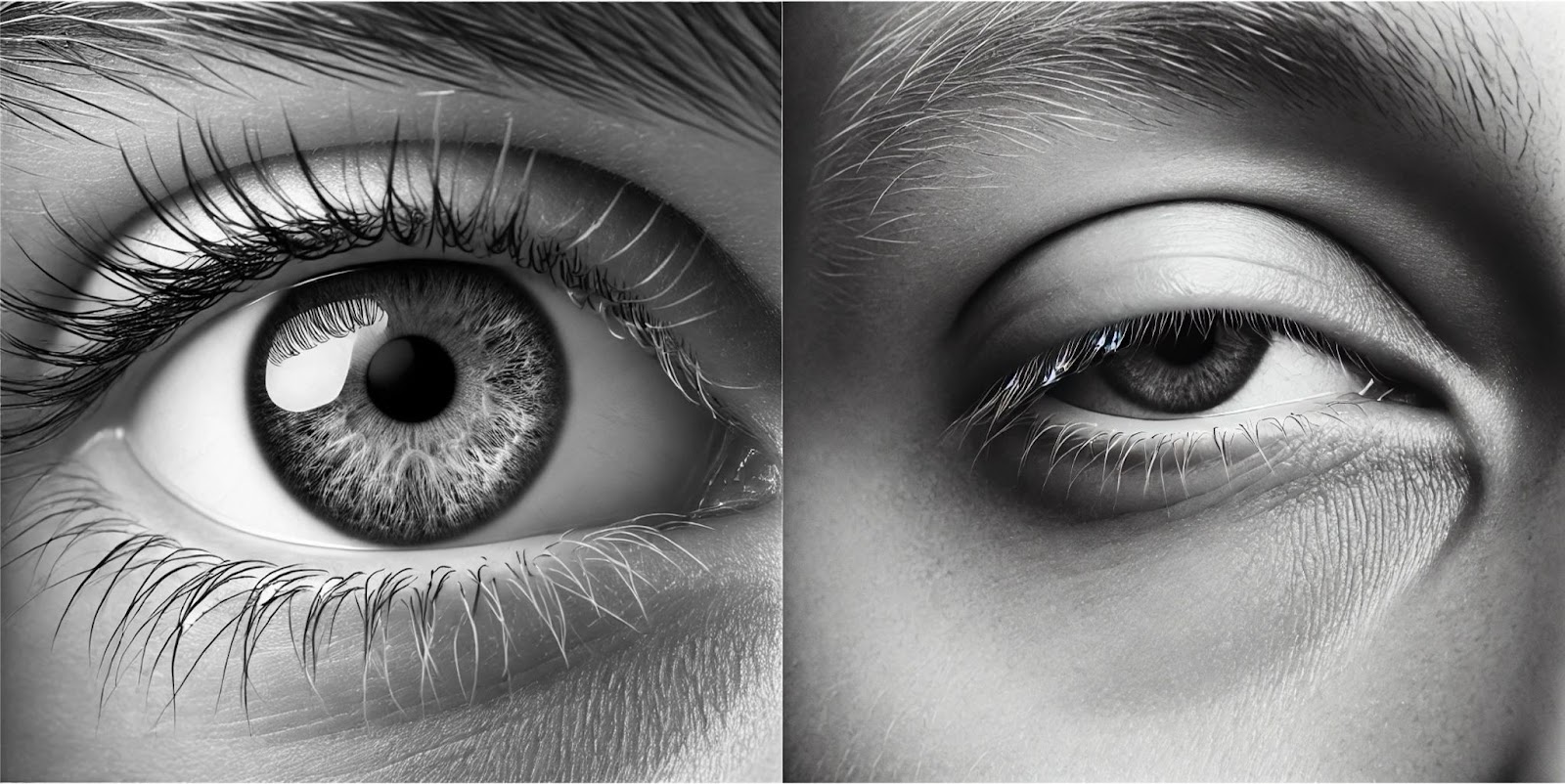
Fig.1 Eye openness is used by humans and AI to determine drowsiness
Human observers detect drowsiness based on eye openness (among other factors) and the AI can be trained to do exactly the same - relying on percentage closure and other similar metrics that characterize how much of the eye is open.
While Drowsiness 1.0 solely focused on eye closure duration statistics, our analysis of extensive data has led us to enhance this approach. Drowsiness 2.0 is based on multiple features. In addition to eye openness, additional features such as blink rate, blink duration, eye closure and statistics derived from these measurements are used to decide if the driver is drowsy or fatigued with a very high accuracy.
Alerting drivers at the earliest signs of drowsiness
It is highly desirable to alert drivers before the eyes close or are nearly closed - detecting the early signs of fatigue or drowsiness is very challenging, especially when one does not want false positives.
Using blink statistics, it is possible to differentiate between a regular state and a driver being drowsy.
Figure 2 illustrates the early stages of drowsiness, which are characterized by short eye closure statistics. Identifying the transitions from slow eye open to close, as well as from slow close to open, is crucial for accurately characterizing early drowsiness. By focusing on these transitions, we can potentially detect drowsiness in its initial stages. This allows for timely alerts well before the driver falls asleep.

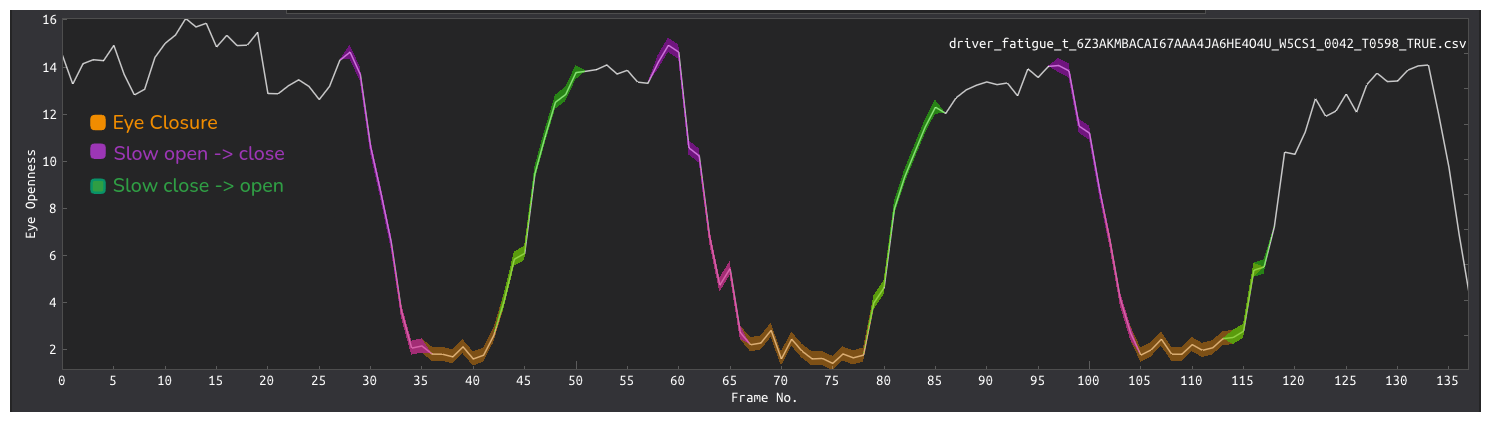
Fig.2 Detecting early stages of drowsiness based on blink analysis
Detecting when a driver is struggling to stay alert
Frequent eye blinks, especially those lasting longer than normal, are a reliable predictor of drowsiness Figure 3 illustrates one such example. These blinks can serve as indicators, additionally when combined with eye closure and eye open <-> close transitions in future windows to predict drowsy confidently
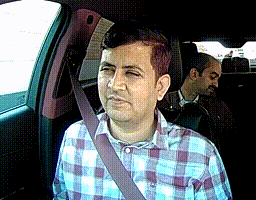

Fig.3 Illustration of frequent blinks pattern during early stages of sleep
Fusing the multiple features
While extracting features is incredibly challenging, there is still a lot more processing needed to extract accurate insights on driver state. Various preprocessing stages to filter out noise, incorporating additional metadata such as head pose, landmarks, etc. ensure further refinement of the features. Multiple statistical measures including frequency, duration, and magnitude, and the relation between these features are used to assign weights to the features according to different configured parameters to establish the confidence level of the event. This ensures a very high precision (low false positives), while maintaining good recall (low false negatives).
Drowsiness 2.0 - alerting drivers before they fall asleep
In conclusion, our latest update, **Drowsiness 2.0**, represents a significant leap forward in preventing drowsy driving incidents. By detecting the early signs of fatigue more accurately than ever before, we can alert drivers before they reach the critical stage of actually falling asleep at the wheel. This proactive approach not only enhances driver safety but also reduces the risk of accidents, protects fleet assets, and lowers operational costs related to crashes. With real-time, in-cabin alerts, Drowsiness 2.0 ensures that drivers can stay alert and safe on the road, reinforcing our commitment to helping commercial fleets operate more efficiently and securely.
To learn more about Drowsiness 2.0 and the benefits of RideView for fleets you work with, please reach out to contact@lightmetrics.co